Datasets:
File size: 15,664 Bytes
b54d910 3bf0d4f b54d910 112765a |
1 2 3 4 5 6 7 8 9 10 11 12 13 14 15 16 17 18 19 20 21 22 23 24 25 26 27 28 29 30 31 32 33 34 35 36 37 38 39 40 41 42 43 44 45 46 47 48 49 50 51 52 53 54 55 56 57 58 59 60 61 62 63 64 65 66 67 68 69 70 71 72 73 74 75 76 77 78 79 80 81 82 83 84 85 86 87 88 89 90 91 92 93 94 95 96 97 98 99 100 101 102 103 104 105 106 107 108 109 110 111 112 113 114 115 116 117 118 119 120 121 122 123 124 125 126 127 128 129 130 131 132 133 134 135 136 137 138 139 140 141 142 143 144 145 146 147 148 149 150 151 152 153 154 155 156 157 158 159 160 161 162 163 164 165 166 167 168 169 170 171 172 173 174 175 176 177 178 179 180 181 182 183 184 185 186 187 188 189 190 191 192 193 194 195 196 197 198 199 200 201 202 203 204 205 206 207 208 209 210 211 212 213 214 215 216 217 218 219 220 221 222 223 224 225 226 227 228 229 230 231 232 233 234 235 236 237 238 239 240 241 242 243 244 245 246 247 248 249 250 251 252 253 254 255 256 257 258 259 260 261 262 263 264 265 266 267 268 269 270 271 272 273 274 275 276 277 278 279 280 281 282 283 284 285 286 287 288 289 290 291 292 293 294 295 296 297 298 299 300 301 302 303 304 305 306 307 308 309 310 311 312 313 314 315 316 317 318 319 320 321 322 323 324 325 326 327 328 329 330 331 332 333 334 335 336 337 338 339 340 341 342 343 344 345 346 347 348 349 350 351 352 353 354 355 356 357 358 359 360 |
---
viewer: false
annotations_creators:
- expert-generated
language: []
language_creators:
- expert-generated
license:
- cc-by-nc-4.0
multilinguality:
- monolingual
pretty_name: "Embrapa Wine Grape Instance Segmentation Dataset \u2013 Embrapa WGISD "
size_categories:
- 1K<n<10K
source_datasets:
- original
tags:
- agriculture
- viticulture
- fruit detection
task_categories:
- object-detection
task_ids: []
---
Embrapa Wine Grape Instance Segmentation Dataset – Embrapa WGISD
================================================================
[](https://zenodo.org/badge/latestdoi/199083745)
This is a detailed description of the dataset, a
*datasheet for the dataset* as proposed by [Gebru *et al.*](https://arxiv.org/abs/1803.09010)
Motivation for Dataset Creation
-------------------------------
### Why was the dataset created?
Embrapa WGISD (*Wine Grape Instance Segmentation Dataset*) was created
to provide images and annotation to study *object detection and instance
segmentation* for image-based monitoring and field robotics in
viticulture. It provides instances from five different grape varieties
taken on field. These instances shows variance in grape pose,
illumination and focus, including genetic and phenological variations
such as shape, color and compactness.
### What (other) tasks could the dataset be used for?
Possible uses include relaxations of the instance segmentation problem:
classification (Is a grape in the image?), semantic segmentation (What
are the "grape pixels" in the image?), object detection (Where are
the grapes in the image?), and counting (How many berries are there
per cluster?). The WGISD can also be used in grape variety
identification.
### Who funded the creation of the dataset?
The building of the WGISD dataset was supported by the Embrapa SEG
Project 01.14.09.001.05.04, *Image-based metrology for Precision
Agriculture and Phenotyping*, and the CNPq PIBIC Program (grants
161165/2017-6 and 125044/2018-6).
Dataset Composition
-------------------
### What are the instances?
Each instance consists in a RGB image and an annotation describing grape
clusters locations as bounding boxes. A subset of the instances also
contains binary masks identifying the pixels belonging to each grape
cluster. Each image presents at least one grape cluster. Some grape
clusters can appear far at the background and should be ignored.
### Are relationships between instances made explicit in the data?
File names prefixes identify the variety observed in the instance.
| Prefix | Variety |
| --- | --- |
| CDY | *Chardonnay* |
| CFR | *Cabernet Franc* |
| CSV | *Cabernet Sauvignon*|
| SVB | *Sauvignon Blanc* |
| SYH | *Syrah* |
### How many instances of each type are there?
The dataset consists of 300 images containing 4,432 grape clusters
identified by bounding boxes. A subset of 137 images also contains
binary masks identifying the pixels of each cluster. It means that from
the 4,432 clusters, 2,020 of them presents binary masks for instance
segmentation, as summarized in the following table.
|Prefix | Variety | Date | Images | Boxed clusters | Masked clusters|
| --- | --- | --- | --- | --- | --- |
|CDY | *Chardonnay* | 2018-04-27 | 65 | 840 | 308|
|CFR | *Cabernet Franc* | 2018-04-27 | 65 | 1,069 | 513|
|CSV | *Cabernet Sauvignon* | 2018-04-27 | 57 | 643 | 306|
|SVB | *Sauvignon Blanc* | 2018-04-27 | 65 | 1,316 | 608|
|SYH | *Syrah* | 2017-04-27 | 48 | 563 | 285|
|Total | | | 300 | 4,431 | 2,020|
*General information about the dataset: the grape varieties and the associated identifying prefix, the date of image capture on field, number of images (instances) and the identified grapes clusters.*
#### Contributions
Another subset of 111 images with separated and non-occluded grape
clusters was annotated with point annotations for every berry by F. Khoroshevsky and S. Khoroshevsky ([Khoroshevsky *et al.*, 2021](https://doi.org/10.1007/978-3-030-65414-6_19)). Theses annotations are available in `test_berries.txt` , `train_berries.txt` and `val_berries.txt`
|Prefix | Variety | Berries |
| --- | --- | --- |
|CDY | *Chardonnay* | 1,102 |
|CFR | *Cabernet Franc* | 1,592 |
|CSV | *Cabernet Sauvignon* | 1,712 |
|SVB | *Sauvignon Blanc* | 1,974 |
|SYH | *Syrah* | 969 |
|Total | | 7,349 |
*Berries annotations by F. Khoroshevsky and S. Khoroshevsky.*
Geng Deng ([Deng *et al.*, 2020](https://doi.org/10.1007/978-3-030-63820-7_66))
provided point-based annotations for berries in all 300 images, summing 187,374 berries.
These annotations are available in `contrib/berries`.
Daniel Angelov (@23pointsNorth) provided a version for the annotations in [COCO format](https://cocodataset.org/#format-data). See `coco_annotations` directory.
### What data does each instance consist of?
Each instance contains a 8-bits RGB image and a text file containing one
bounding box description per line. These text files follows the "YOLO
format"
CLASS CX CY W H
*class* is an integer defining the object class – the dataset presents
only the grape class that is numbered 0, so every line starts with this
“class zero” indicator. The center of the bounding box is the point
*(c_x, c_y)*, represented as float values because this format normalizes
the coordinates by the image dimensions. To get the absolute position,
use *(2048 c_x, 1365 c_y)*. The bounding box dimensions are
given by *W* and *H*, also normalized by the image size.
The instances presenting mask data for instance segmentation contain
files presenting the `.npz` extension. These files are compressed
archives for NumPy $n$-dimensional arrays. Each array is a
*H X W X n_clusters* three-dimensional array where
*n_clusters* is the number of grape clusters observed in the
image. After assigning the NumPy array to a variable `M`, the mask for
the *i*-th grape cluster can be found in `M[:,:,i]`. The *i*-th mask
corresponds to the *i*-th line in the bounding boxes file.
The dataset also includes the original image files, presenting the full
original resolution. The normalized annotation for bounding boxes allows
easy identification of clusters in the original images, but the mask
data will need to be properly rescaled if users wish to work on the
original full resolution.
#### Contributions
*For `test_berries.txt` , `train_berries.txt` and `val_berries.txt`*:
The berries annotations are following a similar notation with the only
exception being that each text file (train/val/test) includes also the
instance file name.
FILENAME CLASS CX CY
where *filename* stands for instance file name, *class* is an integer
defining the object class (0 for all instances) and the point *(c_x, c_y)*
indicates the absolute position of each "dot" indicating a single berry in
a well defined cluster.
*For `contrib/berries`*:
The annotations provide the *(x, y)* point position for each berry center, in a tabular form:
X Y
These point-based annotations can be easily loaded using, for example, `numpy.loadtxt`. See `WGISD.ipynb`for examples.
[Daniel Angelov (@23pointsNorth)](https://github.com/23pointsNorth) provided a version for the annotations in [COCO format](https://cocodataset.org/#format-data). See `coco_annotations` directory. Also see [COCO format](https://cocodataset.org/#format-data) for the JSON-based format.
### Is everything included or does the data rely on external resources?
Everything is included in the dataset.
### Are there recommended data splits or evaluation measures?
The dataset comes with specified train/test splits. The splits are found
in lists stored as text files. There are also lists referring only to
instances presenting binary masks.
| | Images | Boxed clusters | Masked clusters |
| ---------------------| -------- | ---------------- | ----------------- |
| Training/Validation | 242 | 3,581 | 1,612 |
| Test | 58 | 850 | 408 |
| Total | 300 | 4,431 | 2,020 |
*Dataset recommended split.*
Standard measures from the information retrieval and computer vision
literature should be employed: precision and recall, *F1-score* and
average precision as seen in [COCO](http://cocodataset.org)
and [Pascal VOC](http://host.robots.ox.ac.uk/pascal/VOC).
### What experiments were initially run on this dataset?
The first experiments run on this dataset are described in [*Grape detection, segmentation and tracking using deep neural networks and three-dimensional association*](https://arxiv.org/abs/1907.11819) by Santos *et al.*. See also the following video demo:
[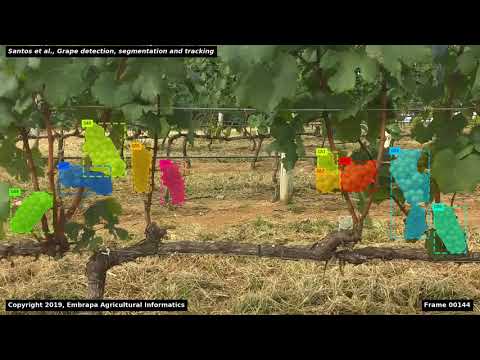](http://www.youtube.com/watch?v=1Hji3GS4mm4 "Grape detection, segmentation and tracking")
**UPDATE**: The JPG files corresponding to the video frames in the [video demo](http://www.youtube.com/watch?v=1Hji3GS4mm4) are now available in the `extras` directory.
Data Collection Process
-----------------------
### How was the data collected?
Images were captured at the vineyards of Guaspari Winery, located at
Espírito Santo do Pinhal, São Paulo, Brazil (Lat -22.181018, Lon
-46.741618). The winery staff performs dual pruning: one for shaping
(after previous year harvest) and one for production, resulting in
canopies of lower density. The image capturing was realized in April
2017 for *Syrah* and in April 2018 for the other varieties.
A Canon EOS REBEL T3i DSLR camera and a Motorola Z2 Play smartphone were
used to capture the images. The cameras were located between the vines
lines, facing the vines at distances around 1-2 meters. The EOS REBEL
T3i camera captured 240 images, including all *Syrah* pictures. The Z2
smartphone grabbed 60 images covering all varieties except *Syrah* . The
REBEL images were scaled to *2048 X 1365* pixels and the Z2 images
to *2048 X 1536* pixels. More data about the capture process can be found
in the Exif data found in the original image files, included in the dataset.
### Who was involved in the data collection process?
T. T. Santos, A. A. Santos and S. Avila captured the images in
field. T. T. Santos, L. L. de Souza and S. Avila performed the
annotation for bounding boxes and masks.
### How was the data associated with each instance acquired?
The rectangular bounding boxes identifying the grape clusters were
annotated using the [`labelImg` tool](https://github.com/tzutalin/labelImg).
The clusters can be under
severe occlusion by leaves, trunks or other clusters. Considering the
absence of 3-D data and on-site annotation, the clusters locations had
to be defined using only a single-view image, so some clusters could be
incorrectly delimited.
A subset of the bounding boxes was selected for mask annotation, using a
novel tool developed by the authors and presented in this work. This
interactive tool lets the annotator mark grape and background pixels
using scribbles, and a graph matching algorithm developed by [Noma *et al.*](https://doi.org/10.1016/j.patcog.2011.08.017)
is employed to perform image segmentation to every pixel in the bounding
box, producing a binary mask representing grape/background
classification.
#### Contributions
A subset of the bounding boxes of well-defined (separated and non-occluded
clusters) was used for "dot" (berry) annotations of each grape to
serve for counting applications as described in [Khoroshevsky *et
al.*](https://doi.org/10.1007/978-3-030-65414-6_19). The berries
annotation was performed by F. Khoroshevsky and S. Khoroshevsky.
Geng Deng ([Deng *et al.*, 2020](https://doi.org/10.1007/978-3-030-63820-7_66))
provided point-based annotations for berries in all 300 images, summing
187,374 berries. These annotations are available in `contrib/berries`.
Deng *et al.* employed [Huawei ModelArt](https://www.huaweicloud.com/en-us/product/modelarts.html),
for their annotation effort.
Data Preprocessing
------------------
### What preprocessing/cleaning was done?
The following steps were taken to process the data:
1. Bounding boxes were annotated for each image using the `labelImg`
tool.
2. Images were resized to *W = 2048* pixels. This resolution proved to
be practical to mask annotation, a convenient balance between grape
detail and time spent by the graph-based segmentation algorithm.
3. A randomly selected subset of images were employed on mask
annotation using the interactive tool based on graph matching.
4. All binaries masks were inspected, in search of pixels attributed to
more than one grape cluster. The annotator assigned the disputed
pixels to the most likely cluster.
5. The bounding boxes were fitted to the masks, which provided a fine
tuning of grape clusters locations.
### Was the “raw” data saved in addition to the preprocessed data?
The original resolution images, containing the Exif data provided by the
cameras, is available in the dataset.
Dataset Distribution
--------------------
### How is the dataset distributed?
The dataset is [available at GitHub](https://github.com/thsant/wgisd).
### When will the dataset be released/first distributed?
The dataset was released in July, 2019.
### What license (if any) is it distributed under?
The data is released under [**Creative Commons BY-NC 4.0 (Attribution-NonCommercial 4.0 International license)**](https://creativecommons.org/licenses/by-nc/4.0/).
There is a request to cite the corresponding paper if the dataset is used. For
commercial use, contact Embrapa Agricultural Informatics business office.
### Are there any fees or access/export restrictions?
There are no fees or restrictions. For commercial use, contact Embrapa
Agricultural Informatics business office.
Dataset Maintenance
-------------------
### Who is supporting/hosting/maintaining the dataset?
The dataset is hosted at Embrapa Agricultural Informatics and all
comments or requests can be sent to [Thiago T. Santos](https://github.com/thsant)
(maintainer).
### Will the dataset be updated?
There is no scheduled updates.
* In May, 2022, [Daniel Angelov (@23pointsNorth)](https://github.com/23pointsNorth) provided a version for the annotations in [COCO format](https://cocodataset.org/#format-data). See `coco_annotations` directory.
* In February, 2021, F. Khoroshevsky and S. Khoroshevsky provided the first extension: the berries ("dot")
annotations.
* In April, 2021, Geng Deng provided point annotations for berries. T. Santos converted Deng's XML files to
easier-to-load text files now available in `contrib/berries` directory.
In case of further updates, releases will be properly tagged at GitHub.
### If others want to extend/augment/build on this dataset, is there a mechanism for them to do so?
Contributors should contact the maintainer by e-mail.
### No warranty
The maintainers and their institutions are *exempt from any liability,
judicial or extrajudicial, for any losses or damages arising from the
use of the data contained in the image database*.
|