๐ CoDe: Collaborative Decoding Makes Visual Auto-Regressive Modeling Efficient
Collaborative Decoding Makes Visual Auto-Regressive Modeling Efficient
Zigeng Chen, Xinyin Ma, Gongfan Fang, Xinchao Wang
Learning and Vision Lab, National University of Singapore
๐ฅฏ[Paper]๐[Project Page] ๐ป [GitHub]

We partition the multi-scale inference process into a seamless collaboration between a large model and a small model.
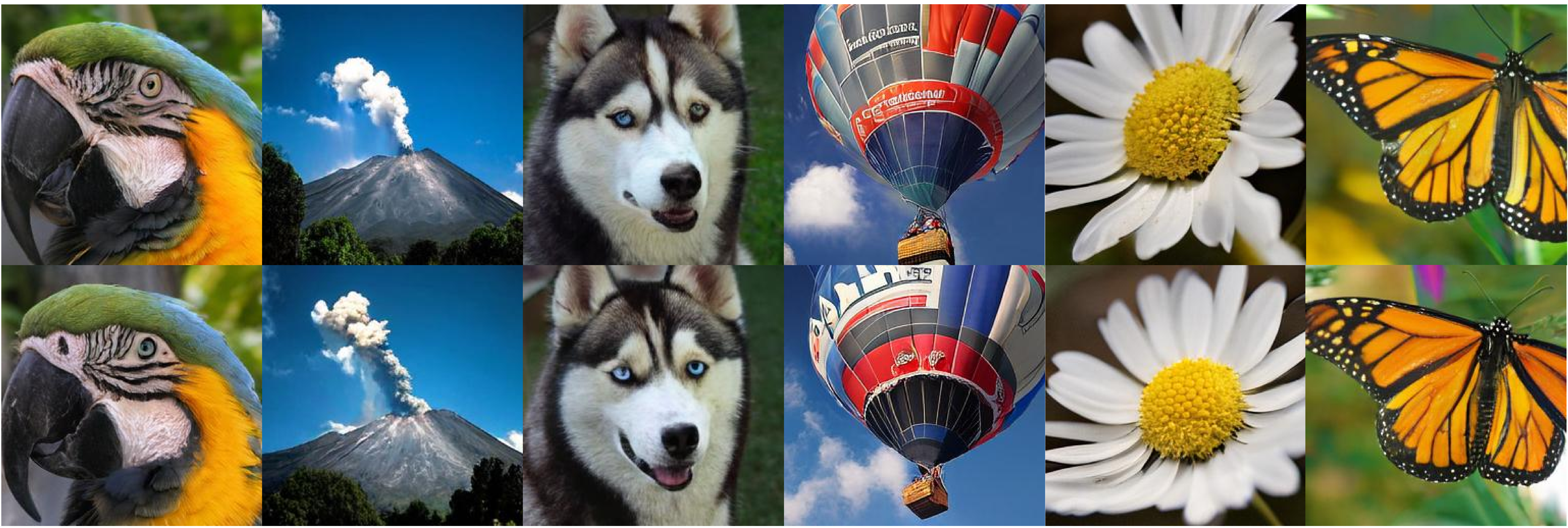
1.7x Speedup and 0.5x memory consumption on ImageNet-256 generation. Top: original VAR-d30; Bottom: CoDe N=8. Speed โโmeasurement does not include vae decoder
๐ก Introduction
We propose Collaborative Decoding (CoDe), a novel decoding strategy tailored to the VAR framework. CoDe capitalizes on two critical observations: the substantially reduced parameter demands at larger scales and the exclusive generation patterns across different scales. Based on these insights, we partition the multi-scale inference process into a seamless collaboration between a large model and a small model.This collaboration yields remarkable efficiency with minimal impact on quality: CoDe achieves a 1.7x speedup, slashes memory usage by around 50%, and preserves image quality with only a negligible FID increase from 1.95 to 1.98. When drafting steps are further decreased, CoDe can achieve an impressive 2.9x acceleration, reaching over 41 images/s at 256x256 resolution on a single NVIDIA 4090 GPU, while preserving a commendable FID of 2.27.