File size: 3,437 Bytes
f2e3e11 ba8375a 39b034d ba8375a f2e3e11 ba8375a 39b034d 1de650d 39b034d 725aa53 43986e7 4654201 725aa53 39b034d 159ae46 39b034d 02493ce 39b034d d0ee505 39b034d d0ee505 44024ec 39b034d |
1 2 3 4 5 6 7 8 9 10 11 12 13 14 15 16 17 18 19 20 21 22 23 24 25 26 27 28 29 30 31 32 33 34 35 36 37 38 39 40 41 42 43 44 45 46 47 48 49 50 51 52 53 54 55 56 57 58 59 60 61 62 63 64 65 66 67 68 69 70 71 |
---
license: apache-2.0
datasets:
- Yirany/UniMM-Chat
- HaoyeZhang/RLHF-V-Dataset
language:
- en
library_name: transformers
---
# Model Card for RLHF-V
[Project Page](https://rlhf-v.github.io/) | [GitHub ](https://github.com/RLHF-V/RLHF-V) | [Demo](http://120.92.209.146:8081/) | [Paper](https://arxiv.org/abs/2312.00849)
## News
* [2024.05.28] π Our RLAIF-V paper is accesible at [arxiv](https://arxiv.org/abs/2405.17220) now!
* [2024.05.20] π We introduce [RLAIF-V](https://github.com/RLHF-V/RLAIF-V), our new alignment framework that utilize open-source models for feedback generation and reach **super GPT-4V trustworthiness**. You can download the corresponding [dataset](https://huggingface.co/datasets/openbmb/RLAIF-V-Dataset) and models ([7B](https://huggingface.co/openbmb/RLAIF-V-7B), [12B](https://huggingface.co/openbmb/RLAIF-V-12B)) now!
* [2024.04.11] π₯ Our data is used in [MiniCPM-V 2.0](https://huggingface.co/openbmb/MiniCPM-V-2), an **end-side** multimodal large language model that exhibits **comparable trustworthiness with GPT-4V**!
## Brief Introduction
RLHF-V is an open-source multimodal large language model with the **lowest hallucination rate** on both long-form instructions and short-form questions.
RLHF-V is trained on [RLHF-V-Dataset](https://huggingface.co/datasets/HaoyeZhang/RLHF-V-Dataset), which contains **fine-grained segment-level human corrections** on diverse instructions. The base model is trained on [UniMM-Chat](https://huggingface.co/datasets/Yirany/UniMM-Chat), which is a high-quality knowledge-intensive SFT dataset. We introduce a new method **Dense Direct Preference Optimization (DDPO)** that can make better use of the fine-grained annotations.
For more details, please refer to our [paper](https://arxiv.org/abs/2312.00849).
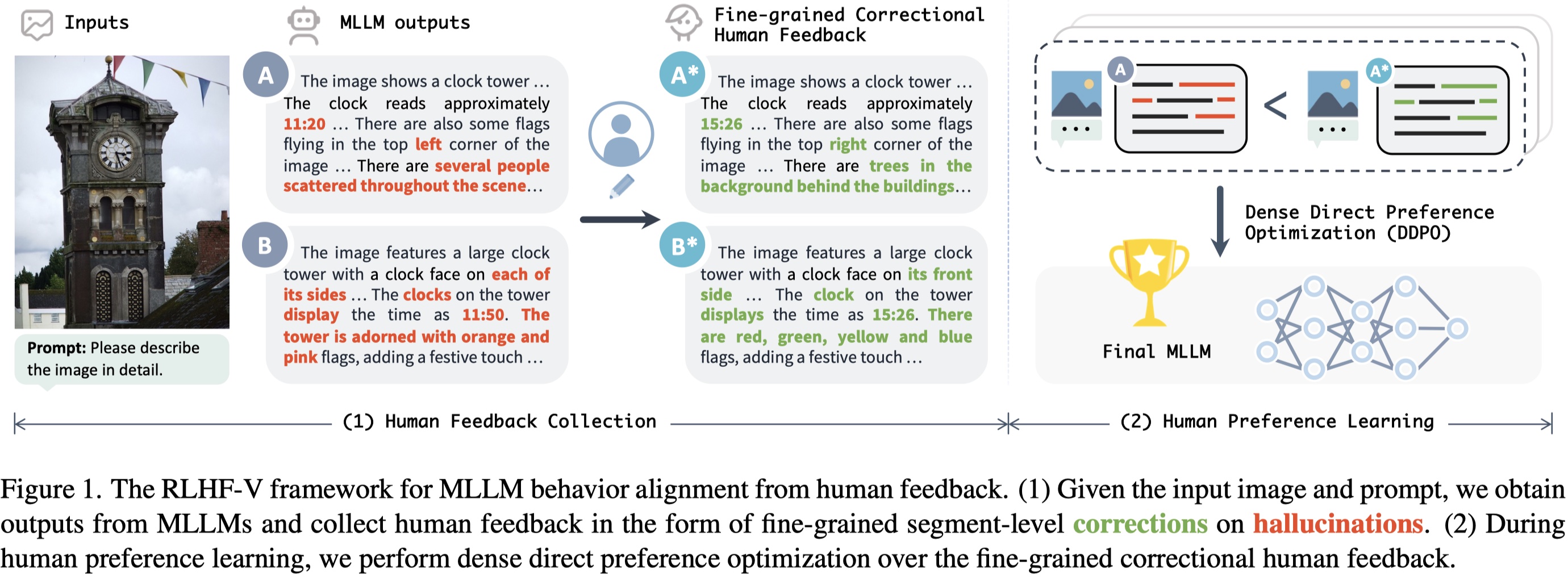
## Model Details
### Model Description
- **Trained from model:** Vicuna-13B
- **Trained on data:** [RLHF-V-Dataset](https://huggingface.co/datasets/HaoyeZhang/RLHF-V-Dataset)
### Model Sources
- **Project Page:** https://rlhf-v.github.io
- **GitHub Repository:** https://github.com/RLHF-V/RLHF-V
- **Demo:** http://120.92.209.146:8081
- **Paper:** https://arxiv.org/abs/2312.00849
## Performance
Low hallucination rate while being informative:

More resistant to over-generalization, even compared to GPT-4V:
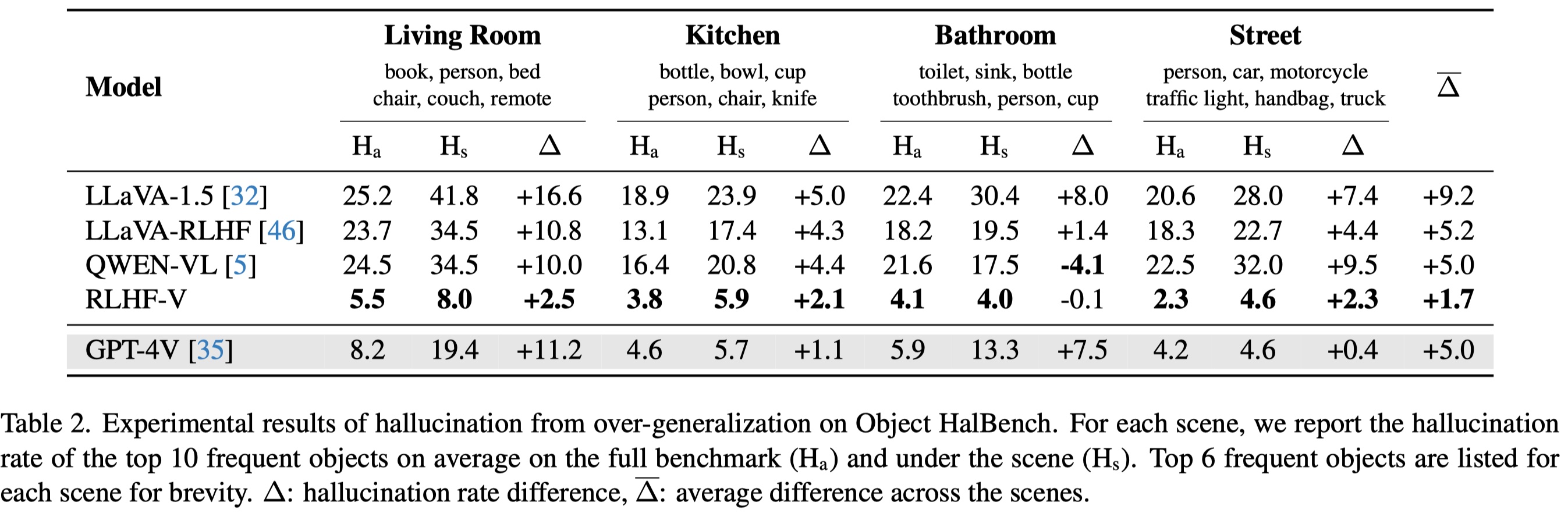
## Citation
If you find this work helpful, please consider cite our papers π:
```bibtex
@article{yu2023rlhf,
title={Rlhf-v: Towards trustworthy mllms via behavior alignment from fine-grained correctional human feedback},
author={Yu, Tianyu and Yao, Yuan and Zhang, Haoye and He, Taiwen and Han, Yifeng and Cui, Ganqu and Hu, Jinyi and Liu, Zhiyuan and Zheng, Hai-Tao and Sun, Maosong and others},
journal={arXiv preprint arXiv:2312.00849},
year={2023}
}
@article{yu2024rlaifv,
title={RLAIF-V: Aligning MLLMs through Open-Source AI Feedback for Super GPT-4V Trustworthiness},
author={Tianyu Yu and Haoye Zhang and Yuan Yao and Yunkai Dang and Da Chen and Xiaoman Lu and Ganqu Cui and Taiwen He and Zhiyuan Liu and Tat-Seng Chua and Maosong Sun},
journal={arXiv preprint arXiv:2405.17220},
year={2024},
}
``` |