|
--- |
|
viewer: false |
|
annotations_creators: |
|
- expert-generated |
|
language: [] |
|
language_creators: |
|
- expert-generated |
|
license: |
|
- cc-by-nc-4.0 |
|
multilinguality: |
|
- monolingual |
|
pretty_name: "Embrapa Wine Grape Instance Segmentation Dataset \u2013 Embrapa WGISD " |
|
size_categories: |
|
- 1K<n<10K |
|
source_datasets: |
|
- original |
|
tags: |
|
- agriculture |
|
- viticulture |
|
- fruit detection |
|
task_categories: |
|
- object-detection |
|
task_ids: [] |
|
--- |
|
|
|
Embrapa Wine Grape Instance Segmentation Dataset – Embrapa WGISD |
|
================================================================ |
|
|
|
[](https://zenodo.org/badge/latestdoi/199083745) |
|
|
|
This is a detailed description of the dataset, a |
|
*datasheet for the dataset* as proposed by [Gebru *et al.*](https://arxiv.org/abs/1803.09010) |
|
|
|
Motivation for Dataset Creation |
|
------------------------------- |
|
|
|
### Why was the dataset created? |
|
|
|
Embrapa WGISD (*Wine Grape Instance Segmentation Dataset*) was created |
|
to provide images and annotation to study *object detection and instance |
|
segmentation* for image-based monitoring and field robotics in |
|
viticulture. It provides instances from five different grape varieties |
|
taken on field. These instances shows variance in grape pose, |
|
illumination and focus, including genetic and phenological variations |
|
such as shape, color and compactness. |
|
|
|
### What (other) tasks could the dataset be used for? |
|
|
|
Possible uses include relaxations of the instance segmentation problem: |
|
classification (Is a grape in the image?), semantic segmentation (What |
|
are the "grape pixels" in the image?), object detection (Where are |
|
the grapes in the image?), and counting (How many berries are there |
|
per cluster?). The WGISD can also be used in grape variety |
|
identification. |
|
|
|
### Who funded the creation of the dataset? |
|
|
|
The building of the WGISD dataset was supported by the Embrapa SEG |
|
Project 01.14.09.001.05.04, *Image-based metrology for Precision |
|
Agriculture and Phenotyping*, and the CNPq PIBIC Program (grants |
|
161165/2017-6 and 125044/2018-6). |
|
|
|
Dataset Composition |
|
------------------- |
|
|
|
### What are the instances? |
|
|
|
Each instance consists in a RGB image and an annotation describing grape |
|
clusters locations as bounding boxes. A subset of the instances also |
|
contains binary masks identifying the pixels belonging to each grape |
|
cluster. Each image presents at least one grape cluster. Some grape |
|
clusters can appear far at the background and should be ignored. |
|
|
|
### Are relationships between instances made explicit in the data? |
|
|
|
File names prefixes identify the variety observed in the instance. |
|
|
|
| Prefix | Variety | |
|
| --- | --- | |
|
| CDY | *Chardonnay* | |
|
| CFR | *Cabernet Franc* | |
|
| CSV | *Cabernet Sauvignon*| |
|
| SVB | *Sauvignon Blanc* | |
|
| SYH | *Syrah* | |
|
|
|
### How many instances of each type are there? |
|
|
|
The dataset consists of 300 images containing 4,432 grape clusters |
|
identified by bounding boxes. A subset of 137 images also contains |
|
binary masks identifying the pixels of each cluster. It means that from |
|
the 4,432 clusters, 2,020 of them presents binary masks for instance |
|
segmentation, as summarized in the following table. |
|
|
|
|Prefix | Variety | Date | Images | Boxed clusters | Masked clusters| |
|
| --- | --- | --- | --- | --- | --- | |
|
|CDY | *Chardonnay* | 2018-04-27 | 65 | 840 | 308| |
|
|CFR | *Cabernet Franc* | 2018-04-27 | 65 | 1,069 | 513| |
|
|CSV | *Cabernet Sauvignon* | 2018-04-27 | 57 | 643 | 306| |
|
|SVB | *Sauvignon Blanc* | 2018-04-27 | 65 | 1,316 | 608| |
|
|SYH | *Syrah* | 2017-04-27 | 48 | 563 | 285| |
|
|Total | | | 300 | 4,431 | 2,020| |
|
|
|
*General information about the dataset: the grape varieties and the associated identifying prefix, the date of image capture on field, number of images (instances) and the identified grapes clusters.* |
|
|
|
#### Contributions |
|
|
|
Another subset of 111 images with separated and non-occluded grape |
|
clusters was annotated with point annotations for every berry by F. Khoroshevsky and S. Khoroshevsky ([Khoroshevsky *et al.*, 2021](https://doi.org/10.1007/978-3-030-65414-6_19)). Theses annotations are available in `test_berries.txt` , `train_berries.txt` and `val_berries.txt` |
|
|
|
|Prefix | Variety | Berries | |
|
| --- | --- | --- | |
|
|CDY | *Chardonnay* | 1,102 | |
|
|CFR | *Cabernet Franc* | 1,592 | |
|
|CSV | *Cabernet Sauvignon* | 1,712 | |
|
|SVB | *Sauvignon Blanc* | 1,974 | |
|
|SYH | *Syrah* | 969 | |
|
|Total | | 7,349 | |
|
|
|
*Berries annotations by F. Khoroshevsky and S. Khoroshevsky.* |
|
Geng Deng ([Deng *et al.*, 2020](https://doi.org/10.1007/978-3-030-63820-7_66)) |
|
provided point-based annotations for berries in all 300 images, summing 187,374 berries. |
|
These annotations are available in `contrib/berries`. |
|
|
|
Daniel Angelov (@23pointsNorth) provided a version for the annotations in [COCO format](https://cocodataset.org/#format-data). See `coco_annotations` directory. |
|
|
|
|
|
### What data does each instance consist of? |
|
|
|
Each instance contains a 8-bits RGB image and a text file containing one |
|
bounding box description per line. These text files follows the "YOLO |
|
format" |
|
|
|
CLASS CX CY W H |
|
|
|
*class* is an integer defining the object class – the dataset presents |
|
only the grape class that is numbered 0, so every line starts with this |
|
“class zero” indicator. The center of the bounding box is the point |
|
*(c_x, c_y)*, represented as float values because this format normalizes |
|
the coordinates by the image dimensions. To get the absolute position, |
|
use *(2048 c_x, 1365 c_y)*. The bounding box dimensions are |
|
given by *W* and *H*, also normalized by the image size. |
|
|
|
The instances presenting mask data for instance segmentation contain |
|
files presenting the `.npz` extension. These files are compressed |
|
archives for NumPy $n$-dimensional arrays. Each array is a |
|
*H X W X n_clusters* three-dimensional array where |
|
*n_clusters* is the number of grape clusters observed in the |
|
image. After assigning the NumPy array to a variable `M`, the mask for |
|
the *i*-th grape cluster can be found in `M[:,:,i]`. The *i*-th mask |
|
corresponds to the *i*-th line in the bounding boxes file. |
|
|
|
The dataset also includes the original image files, presenting the full |
|
original resolution. The normalized annotation for bounding boxes allows |
|
easy identification of clusters in the original images, but the mask |
|
data will need to be properly rescaled if users wish to work on the |
|
original full resolution. |
|
|
|
#### Contributions |
|
|
|
*For `test_berries.txt` , `train_berries.txt` and `val_berries.txt`*: |
|
|
|
The berries annotations are following a similar notation with the only |
|
exception being that each text file (train/val/test) includes also the |
|
instance file name. |
|
|
|
FILENAME CLASS CX CY |
|
|
|
where *filename* stands for instance file name, *class* is an integer |
|
defining the object class (0 for all instances) and the point *(c_x, c_y)* |
|
indicates the absolute position of each "dot" indicating a single berry in |
|
a well defined cluster. |
|
|
|
*For `contrib/berries`*: |
|
|
|
The annotations provide the *(x, y)* point position for each berry center, in a tabular form: |
|
|
|
X Y |
|
|
|
These point-based annotations can be easily loaded using, for example, `numpy.loadtxt`. See `WGISD.ipynb`for examples. |
|
|
|
|
|
[Daniel Angelov (@23pointsNorth)](https://github.com/23pointsNorth) provided a version for the annotations in [COCO format](https://cocodataset.org/#format-data). See `coco_annotations` directory. Also see [COCO format](https://cocodataset.org/#format-data) for the JSON-based format. |
|
|
|
|
|
### Is everything included or does the data rely on external resources? |
|
|
|
Everything is included in the dataset. |
|
|
|
### Are there recommended data splits or evaluation measures? |
|
|
|
The dataset comes with specified train/test splits. The splits are found |
|
in lists stored as text files. There are also lists referring only to |
|
instances presenting binary masks. |
|
|
|
| | Images | Boxed clusters | Masked clusters | |
|
| ---------------------| -------- | ---------------- | ----------------- | |
|
| Training/Validation | 242 | 3,581 | 1,612 | |
|
| Test | 58 | 850 | 408 | |
|
| Total | 300 | 4,431 | 2,020 | |
|
|
|
*Dataset recommended split.* |
|
|
|
|
|
Standard measures from the information retrieval and computer vision |
|
literature should be employed: precision and recall, *F1-score* and |
|
average precision as seen in [COCO](http://cocodataset.org) |
|
and [Pascal VOC](http://host.robots.ox.ac.uk/pascal/VOC). |
|
|
|
### What experiments were initially run on this dataset? |
|
|
|
The first experiments run on this dataset are described in [*Grape detection, segmentation and tracking using deep neural networks and three-dimensional association*](https://arxiv.org/abs/1907.11819) by Santos *et al.*. See also the following video demo: |
|
|
|
[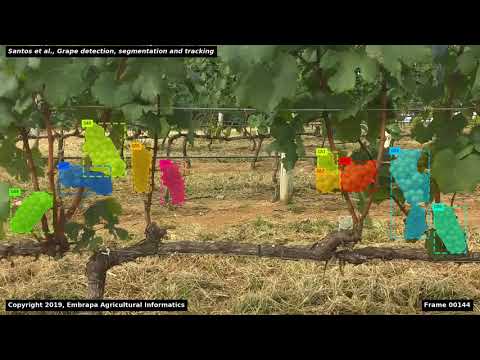](http://www.youtube.com/watch?v=1Hji3GS4mm4 "Grape detection, segmentation and tracking") |
|
|
|
**UPDATE**: The JPG files corresponding to the video frames in the [video demo](http://www.youtube.com/watch?v=1Hji3GS4mm4) are now available in the `extras` directory. |
|
|
|
Data Collection Process |
|
----------------------- |
|
|
|
### How was the data collected? |
|
|
|
Images were captured at the vineyards of Guaspari Winery, located at |
|
Espírito Santo do Pinhal, São Paulo, Brazil (Lat -22.181018, Lon |
|
-46.741618). The winery staff performs dual pruning: one for shaping |
|
(after previous year harvest) and one for production, resulting in |
|
canopies of lower density. The image capturing was realized in April |
|
2017 for *Syrah* and in April 2018 for the other varieties. |
|
|
|
A Canon EOS REBEL T3i DSLR camera and a Motorola Z2 Play smartphone were |
|
used to capture the images. The cameras were located between the vines |
|
lines, facing the vines at distances around 1-2 meters. The EOS REBEL |
|
T3i camera captured 240 images, including all *Syrah* pictures. The Z2 |
|
smartphone grabbed 60 images covering all varieties except *Syrah* . The |
|
REBEL images were scaled to *2048 X 1365* pixels and the Z2 images |
|
to *2048 X 1536* pixels. More data about the capture process can be found |
|
in the Exif data found in the original image files, included in the dataset. |
|
|
|
### Who was involved in the data collection process? |
|
|
|
T. T. Santos, A. A. Santos and S. Avila captured the images in |
|
field. T. T. Santos, L. L. de Souza and S. Avila performed the |
|
annotation for bounding boxes and masks. |
|
|
|
### How was the data associated with each instance acquired? |
|
|
|
The rectangular bounding boxes identifying the grape clusters were |
|
annotated using the [`labelImg` tool](https://github.com/tzutalin/labelImg). |
|
The clusters can be under |
|
severe occlusion by leaves, trunks or other clusters. Considering the |
|
absence of 3-D data and on-site annotation, the clusters locations had |
|
to be defined using only a single-view image, so some clusters could be |
|
incorrectly delimited. |
|
|
|
A subset of the bounding boxes was selected for mask annotation, using a |
|
novel tool developed by the authors and presented in this work. This |
|
interactive tool lets the annotator mark grape and background pixels |
|
using scribbles, and a graph matching algorithm developed by [Noma *et al.*](https://doi.org/10.1016/j.patcog.2011.08.017) |
|
is employed to perform image segmentation to every pixel in the bounding |
|
box, producing a binary mask representing grape/background |
|
classification. |
|
|
|
#### Contributions |
|
|
|
A subset of the bounding boxes of well-defined (separated and non-occluded |
|
clusters) was used for "dot" (berry) annotations of each grape to |
|
serve for counting applications as described in [Khoroshevsky *et |
|
al.*](https://doi.org/10.1007/978-3-030-65414-6_19). The berries |
|
annotation was performed by F. Khoroshevsky and S. Khoroshevsky. |
|
|
|
Geng Deng ([Deng *et al.*, 2020](https://doi.org/10.1007/978-3-030-63820-7_66)) |
|
provided point-based annotations for berries in all 300 images, summing |
|
187,374 berries. These annotations are available in `contrib/berries`. |
|
Deng *et al.* employed [Huawei ModelArt](https://www.huaweicloud.com/en-us/product/modelarts.html), |
|
for their annotation effort. |
|
|
|
Data Preprocessing |
|
------------------ |
|
|
|
### What preprocessing/cleaning was done? |
|
|
|
The following steps were taken to process the data: |
|
|
|
1. Bounding boxes were annotated for each image using the `labelImg` |
|
tool. |
|
|
|
2. Images were resized to *W = 2048* pixels. This resolution proved to |
|
be practical to mask annotation, a convenient balance between grape |
|
detail and time spent by the graph-based segmentation algorithm. |
|
|
|
3. A randomly selected subset of images were employed on mask |
|
annotation using the interactive tool based on graph matching. |
|
|
|
4. All binaries masks were inspected, in search of pixels attributed to |
|
more than one grape cluster. The annotator assigned the disputed |
|
pixels to the most likely cluster. |
|
|
|
5. The bounding boxes were fitted to the masks, which provided a fine |
|
tuning of grape clusters locations. |
|
|
|
### Was the “raw” data saved in addition to the preprocessed data? |
|
|
|
The original resolution images, containing the Exif data provided by the |
|
cameras, is available in the dataset. |
|
|
|
Dataset Distribution |
|
-------------------- |
|
|
|
### How is the dataset distributed? |
|
|
|
The dataset is [available at GitHub](https://github.com/thsant/wgisd). |
|
|
|
### When will the dataset be released/first distributed? |
|
|
|
The dataset was released in July, 2019. |
|
|
|
### What license (if any) is it distributed under? |
|
|
|
The data is released under [**Creative Commons BY-NC 4.0 (Attribution-NonCommercial 4.0 International license)**](https://creativecommons.org/licenses/by-nc/4.0/). |
|
There is a request to cite the corresponding paper if the dataset is used. For |
|
commercial use, contact Embrapa Agricultural Informatics business office. |
|
|
|
### Are there any fees or access/export restrictions? |
|
|
|
There are no fees or restrictions. For commercial use, contact Embrapa |
|
Agricultural Informatics business office. |
|
|
|
Dataset Maintenance |
|
------------------- |
|
|
|
### Who is supporting/hosting/maintaining the dataset? |
|
|
|
The dataset is hosted at Embrapa Agricultural Informatics and all |
|
comments or requests can be sent to [Thiago T. Santos](https://github.com/thsant) |
|
(maintainer). |
|
|
|
### Will the dataset be updated? |
|
|
|
There is no scheduled updates. |
|
|
|
* In May, 2022, [Daniel Angelov (@23pointsNorth)](https://github.com/23pointsNorth) provided a version for the annotations in [COCO format](https://cocodataset.org/#format-data). See `coco_annotations` directory. |
|
|
|
* In February, 2021, F. Khoroshevsky and S. Khoroshevsky provided the first extension: the berries ("dot") |
|
annotations. |
|
|
|
* In April, 2021, Geng Deng provided point annotations for berries. T. Santos converted Deng's XML files to |
|
easier-to-load text files now available in `contrib/berries` directory. |
|
|
|
In case of further updates, releases will be properly tagged at GitHub. |
|
|
|
### If others want to extend/augment/build on this dataset, is there a mechanism for them to do so? |
|
|
|
Contributors should contact the maintainer by e-mail. |
|
|
|
### No warranty |
|
|
|
The maintainers and their institutions are *exempt from any liability, |
|
judicial or extrajudicial, for any losses or damages arising from the |
|
use of the data contained in the image database*. |
|
|
|
|